"With 3 in-house experts, it is key that they can rely on a common tool as reliable and insightful as Seiso."
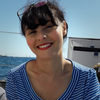
Leila Couailler Moumeni
Acquisition Manager
Webedia
"Finally, an efficient tool to help me develop the non-branded keyword traffic!"
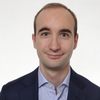
Christophe Gros
CMO
Hast
"Well thought through, convenient and exhaustive, i use Seiso to identify my priorities."
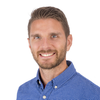
Steeve Belair
Digital Marketing Manager
Casal Sport